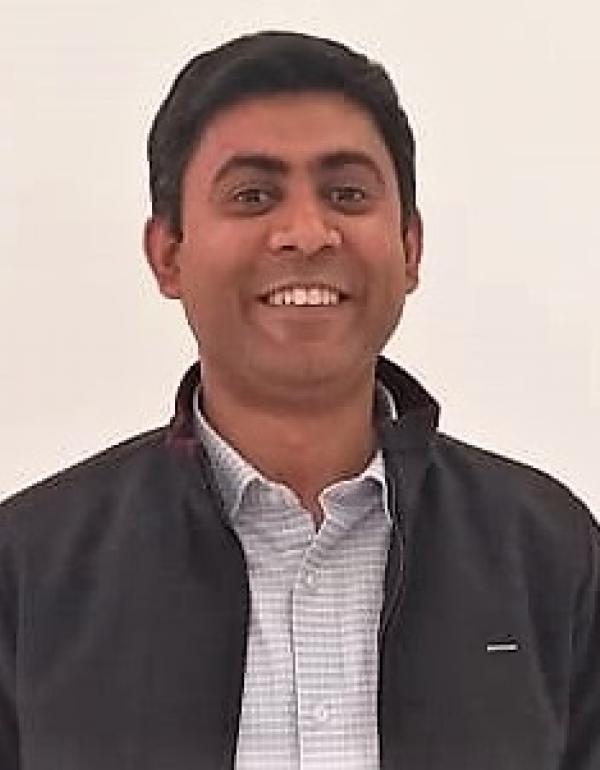
Dr. Ashish Anand is an Associate Professor at the Department of Computer Science and Engineering at IIT Guwahati. He joined the institute in February 2011. His primary research interests are in Natural Language Processing, Clinical and Biomedical Text Mining, Computational Biology and Machine Learning. His research works (with more than 1000 citations) have been published in top-tiered conferences and journals including ACL, EACL, BioNLP, IEEE Transactions on Computational Biology and Bioinformatics. Dr. Anand obtained his Masters (Int-Msc, 5 yrs) in Mathematics and Scientific Computing from Indian Institute of Technology Kanpur. He did his Ph.D. from Nanyang Technological University Singapore on “Computational Intelligence Methods for Problems in Computational Biology." In particular, he worked on multi-class classification, template clustering for short time series data and imbalanced binary classification problems. And before joining IIT G, he was part of European Consortium, BaSySBio at Systems Biology Lab (Group Leader: Dr. Benno Schwikowski) Institut Pasteur, Paris. His post-doc work was mainly concentrated on regulatory network reconstruction and pathway analysis.
Abstract OpenTox Asia 2019
Machine Learning Methods for Drug Repositioning or Drug Repurposing
Drug development is an expensive (estimated average cost: 500 million to 2 billion US $) and time consuming process (estimated time duration: 15 to 20 years). Drug Repositioning or Repurposing (DR) is a complementary approach to classic drug discovery leading to reduction in time and cost in any drug development process. Drug Repositioning is a systematic approach to predict novel medical indications for already approved drugs. One study estimates that 30% of all the drugs which have been approved by the US Food and Drug Administration (FDA) are the result of drug repositioning.
Due to advancement in biological science, large amount of drug-biological effects data has been generated. Using computational models this data can be used to predict new indications for approved drugs. Working principle of all the methods developed for the drug repositioning problem rely on two important aspects related to drugs and diseases. First is drugs often bind to multiple targets resulting into various biological effects including side-effects. Second is a biological target of a drug, which is relevant to a particular disease, may also be directly or indirectly associated with other diseases.
This talk primarily aims to discuss various data-driven and machine learning approaches for drug repositioning. Furthermore, this talk will also discuss methods which try to exploit information available in form of free texts in biomedical research articles.