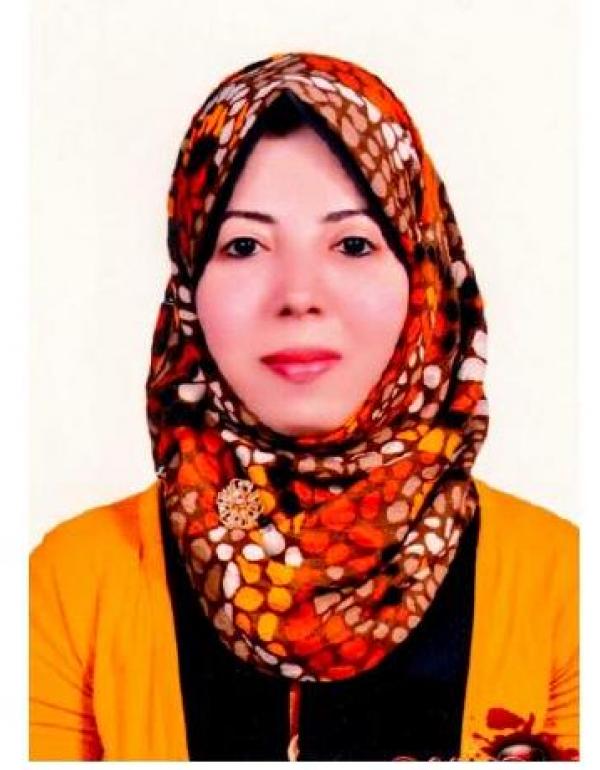
Asmaa Ali is a Research Data Scientist at Edelweiss Connect GmbH, where she specialises in developing intelligent systems for Chemical Risk Assessment. Her research interests encompass the dynamic intersection of public health, translational toxicology, and precision medicine. My passion lies in exploring how these fields can collectively harness the power of cutting-edge research and technology to enhance community health strategies while tailoring medical interventions to the individual needs of patients. Prior to joining Edelweiss Connect, Asmaa held the position of Senior Bioinformatician at the Egypt Center for Research and Regenerative Medicine. In this role, she utilised her expertise in machine learning and data analysis techniques to extract valuable insights from genomics data. Asmaa's professional experience also includes working as a Data Scientist at Rosettastein Consulting GmbH. In this role, she excelled in predictive modelling to optimise models in predictive toxicology. She actively evaluated the effectiveness and accuracy of new data sources and data-gathering methods, continuously enhancing the reliability and validity of predictive models. Additionally, Asmaa played a vital role in developing customised data models and algorithms for diverse datasets, contributing to the improvement of product development, marketing strategies, and business tactics.
OpenTox Virtual Conference 2023
AI-Driven Insights into Chemical-Protein Dynamics: A Multifaceted Approach
In this innovative venture, we explore the use of artificial intelligence (AI) to decode the complex web of chemical-protein interactions. Our goal is to harness the computational might of AI to go beyond what traditional study methods have achieved, bringing to light the subtle interplay of molecules within biological environments. Our scientific journey navigated through a terrain of challenges such as the diversity of chemical compound synonyms, extensive literature surveys, and the intricate task of extracting precise relationships. We meticulously extracted synonyms from a plethora of literature, employing strategic methods to gauge their significance. Our comprehensive literature review spanned two decades of research indexed on PubMed, ensuring that our study is as inclusive as possible of the various terminologies in use.
Methodologically, our approach was two-pronged: comprehensive data collation paired with refined algorithmic analysis. Our initial step involved a diligent collection and ranking of chemical synonyms from various databases, guided by their frequency in literature. We then applied advanced Named Entity Recognition (NER) to not only spot and label chemicals and proteins but also to enhance coreference resolution. This involved mapping out entities that refer to the same concept within the text and analyzing the relationships between these entities to form a cohesive understanding of chemical-protein dynamics. Simultaneously, we developed a rich question-and-answer dataset, designed to reflect the diverse range of potential inquiries in this field and maintain the integrity of the information. This Q&A compilation which was converted later to instructions dataset was instrumental in the fine-tuning process of our large language model (Llama-2), which were carefully adjusted to discern the nuanced aspects of toxicological data.
The outcome to date is a nascent AI model, poised to automate the detection and analysis of chemical-protein interactions. Although it is not yet fully refined, it represents a critical step forward. Enhancements are on the horizon, with plans to integrate more data and refine our algorithms to improve both accuracy and efficiency.
Our aim is to ultimately develop a comprehensive system that can seamlessly sift through extensive scientific literature, providing detailed and reliable insights into chemical toxicity within biological systems. The current progress lays the groundwork for future breakthroughs in toxicological research, with an ongoing commitment to evolving our AI model for more advanced, data-driven solutions.