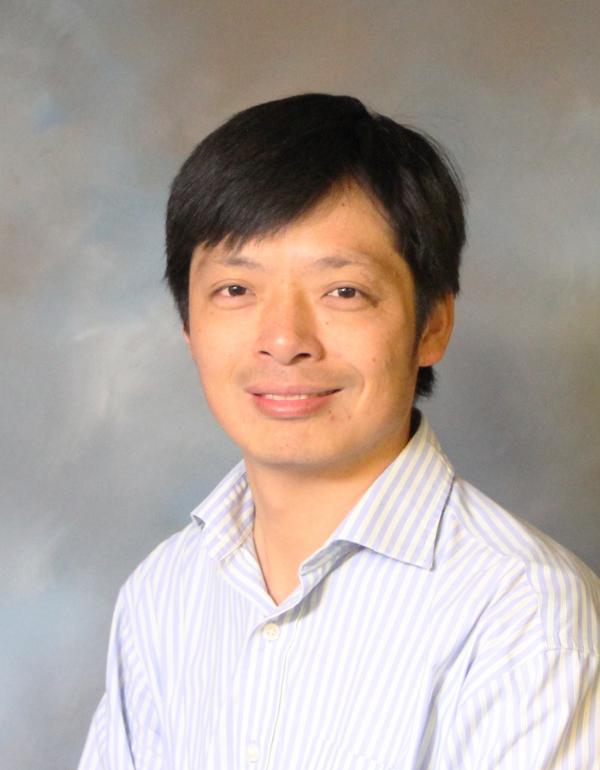
Dr. Minjun Chen is a senior scientist at Division of Bioinformatics and Biostatistics, the US FDA’s National Center for Toxicological Research (NCTR). His major research interests encompass to develop the state-of-the-art computational technologies for the study of drug-induced liver injury and liver disease. He developed the “rule-of-two” and DILIscore models and applied these models to evaluate drug-induced liver injury (DILI) risk of over 50 NDA and IND submissions for supporting the FDA reviewers’ regulatory decision. He also developed the DILIrank database, which has been widely used by the community to support the development of DILI predictive models and biomarkers. He is one of the founders and the chair of the FDA Liver Toxicity Working Group (LTWG) and serves as the vice president of the computational toxicological special section (CTSS) of Society of Toxicology. Dr. Chen has published over 100 peer-reviewed papers and book chapters and is the major editor of a book titled “Drug-Induced
Liver Toxicity” published by Humana Press, Springer in 2018.
OpenTox Virtual Conference 2023
Chair session description - contains an overview of the session including theme and goals
Drug-induced liver injury (DILI) is a serious adverse drug reaction that can lead to acute liver failure and death. It has been one of the most frequent reasons for post-marketing withdrawals over the past 50 years. Many efforts have been invested in de-risking potential human DILI liabilities. Translational methods in hepatotoxicity assessment are used to predict the potential for liver toxicity in humans based on preclinical data. These methods are used to bridge the gap between preclinical and clinical studies. In this session, we will present some recent advances of in silico and in vitro models for predicting DILI in humans, especially those based on adverse outcome pathways (AOPs), aiming to improve the model transparency.