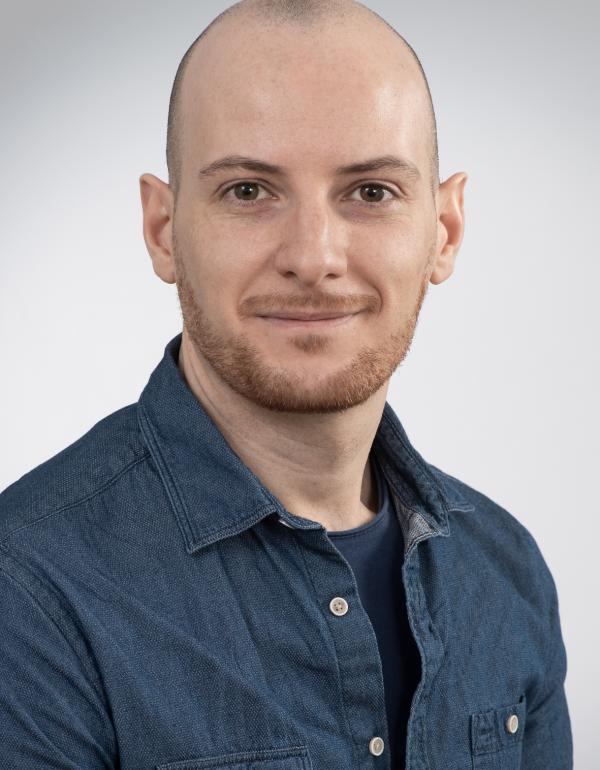
Dr. Fortino holds an MS degree in Computer Science and a Ph.D. in Bioinformatics received from the University of Salerno (Italy). He is currently an Associate Professor, Principal Investigator, and Academy Research Fellow at the University of Eastern Finland, Institute of Biomedicine. Dr. Fortino is leading the Biomedical Informatics research group (https://sites.uef.fi/
OpenTox Virtual Conference 2022
Identifying and prioritizing compounds with endocrine disruption potential
Abstract:
Endocrine disrupting chemicals (EDCs) are a class of chemicals that have the potential to alter the normal functioning of the endocrine system by mimicking, blocking, or interfering with the hormones of the system. Even though there are tools for predicting the endocrine disruption potential of compounds disruption (e.g. ToxCast and ToxPi), none of them is based on toxicogenomics data. We have recently implemented a computational framework to profile the molecular activity of EDCs in an in silico manner and to implement a classification system linking ED exposure to adverse outcomes. Starting from known molecular initiating events of EDCs and using methodologies such as toxicogenomics signatures, gene networks, pathway-based enrichment analysis and machine learning, we built an ensemble predictive model for prioritizing chemicals based on their ED potential, and for inferring associations between EDC exposures and metabolic-related diseases such as atherosclerosis, type 2 diabetes and metabolic syndrome. Benchmark and validation results (based on positive and negative test sets) demonstrate that the proposed method can improve the prediction accuracy of current platforms for EDC screening, such as ToxCast, ToxPi and DEDuCT. Likewise, the provided computational platform can be used to successfully predict metabolic-related diseases linked to EDCs, and to identify novel genes and pathways that are responsive to EDCs.
References:
- Sakhteman, A et al. (2022). Bioinformatics, 38(7), pp.2066-2069.
- Sakhteman, A et al. (2021). Environment International 156 (2021), 106751.