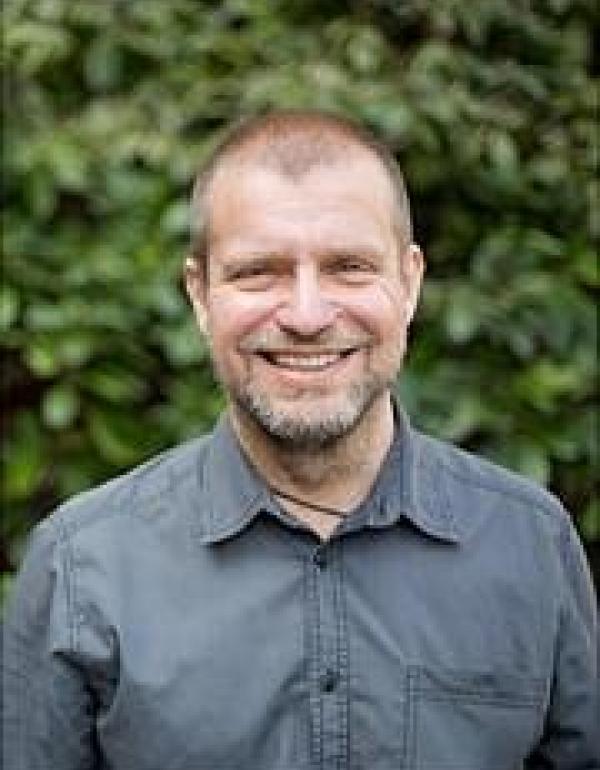
Contact Info
Wojtek Plonka
Senior Scientist at Life Sciences group in FQS Poland (Fujutsu Group)
Wojtek Plonka is a Senior Scientist at Life Sciences group in FQS Poland (Fujutsu Group). He joined Fujitsu short after receiving a degree from Technical Univesrity of Lodz, Poland in the field of Computational Chemistry. He has over 20 years of experience in design, development, and use of software for simulations, particularly in chemistry and drug design. Recently his scientific interests shifted to the area Machine Learning in drug design and chemical safety in joint projects of Fujitsu and Univeristy of Hamburg where he is a Ph.D. student. Designer and coauthor of several software packages including CAChe, SCIGRESS, ADMEWORKS, and others also provides consulting and trainings for both academics and pharma industry.
OpenTox Summer School 2023
- Preprocessing chemical structures for building Structure-Activity Relationship models in Google Colab - Consistent treatment of chemical structures is one of the keys to successful SAR modeling. Issues of reading chemical structures from different file formats, structure cleanup, automatic corrections, tautomer normalization, and conformer generation using open-source Python packages will be discussed. Participants will learn how to create data sets ready for SAR studies with very little programming in Google Colab.
- Building Structure-Activity Relationship models in Google Colab - Advances in Machine Learning techniques allow quick and easy creation of SAR models for predictive purposes. Participants will learn how to build SAR Machine Learning / AI models employing fast and robust fingerprint descriptors using state-of-the-art free open-source Python packages. Issues of model optimization and validation will also be discussed.