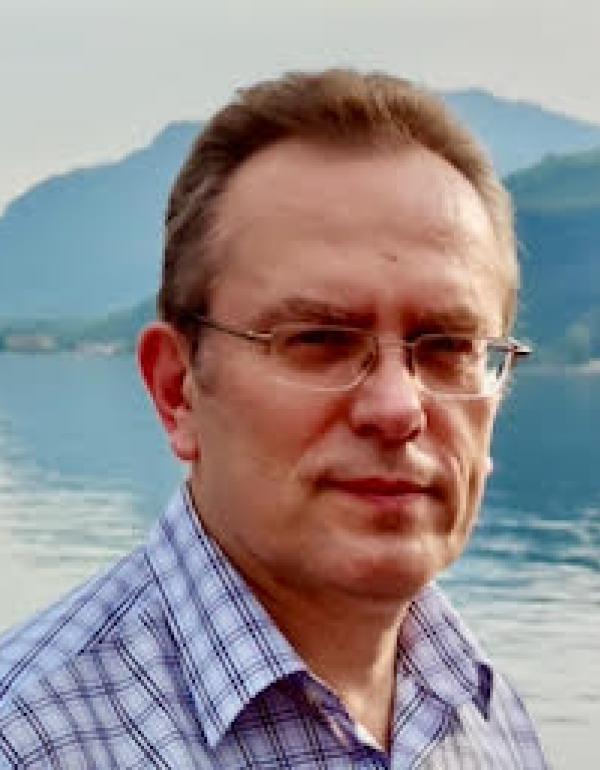
Dr. Igor Tetko received his MSc from Moscow Institute of Physics and Technology (summa cum laude - red diploma), which was one of the top-ranked Universities in the Soviet Union. He did his postdoctoral study at the University of Lausanne in neuroinformatics where he developed algorithms for analysis of EEG data, synfire chains, theoretical modeling of thalamo-cortical organization of brain. Since 2001 Dr. Tetko is at Helmholtz Zentrum München, where he is a group leader in Chemical Informatics. Dr. Tetko co-authored >200 publications in chemoinformatics, bioinformatics, neuroinformatics and machine learning. He coordinated Marie Skłodowska-Curie Innovative Training Network European Industrial Doctorates “Big Data in Chemistry” (http://bigchem.eu), which provided training in development and application of new machine learning algorithms in chemistry and life sciences. He will be a General Chair of International Conference on Artificial Neural Networks (ICANN2024 to be announced soon at https://e-nns.org). Currently he coordinates two Horizon2020 Marie Skłodowska-Curie Innovative Training Network European Industrial Doctorates projects: Advanced machine learning for Innovative Drug Discovery (AIDD) (https://ai-dd.eu) and Explainable AI for Molecules - AiChemist (https://aichemist.eu). He is also an Associate Editor of the ACS ChemResTox (https://pubs.acs.org/journal/
OpenTox Summer School 2023 Abstract
Development of QSAR/QSPR models using representation learning and descriptor based methods using openOCHEM. Which ones are better? (July 24) In a brief overview of OCHEM (https://ochem.eu) and its opensource version (https://github.com/openochem) I will discuss steps required to develop models using this platform. Issue with upload of data, selection of correct units, creation and re-use of existing datasets, development of multiple models and building consensus models will be exemplified. A comparison of traditional and representation learning models and their use as part of consensus models will be discussed. The presentation will be finished with an overview of results of the recent the 1st EUOS/SLAS Joint Challenge: KAGGLE Compound Solubility Challenge https://www.kaggle.com/
- Exercises/Discussion (August 7) this Q&A session will overview modelling results by analyzing performance of different types of methods developed by participants. Analysis of errors (if any) will be provided.