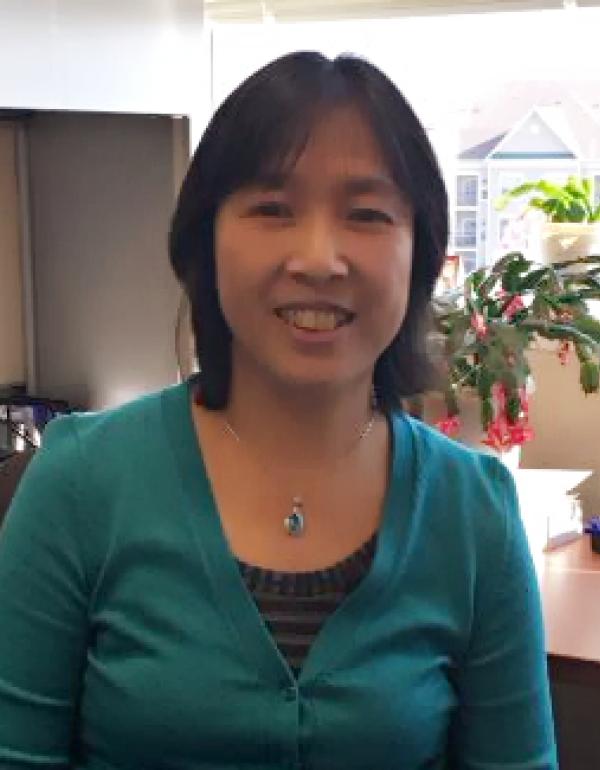
Dr. Ruili Huang is the informatics group leader on the toxicity profiling team at the NIH National Center for Advancing Translational Sciences (NCATS). She also serves as a co-chair of the Tox21 chemical library working group. Dr. Huang and her group contribute to quantitative high-throughput screening (qHTS) data processing and interpretation and development and implementation of software tools and algorithms that facilitate NCATS’ data pipeline. As a computational toxicology team, Dr. Huang’s group evaluates qHTS assay performance for prioritization, analyzes compound in vitro toxicity profiling data to generate hypotheses on compound mechanisms of toxicity, and develops computational models for better prediction of in vivo toxicity. Additionally, her group integrates biological pathway information and qHTS assay data to support interpretation of results. Dr. Huang received her Ph.D. in chemistry from Iowa State University, trained as a computational biologist at the National Cancer Institute, and joined NCATS in 2006.
Abstract for OpenTox Summer School 2023
Predictive Modeling based on Tox21 data
The U.S. Tox21 program has screened a library of approximately 10,000 (10K) structurally diverse environmental chemicals and drugs against a battery of ~80 in vitro assays in a quantitative high throughput screening (qHTS) format, generating over 100 million data points that have been made publicly available. Counter screens are also employed to minimize interferences from non-target specific assay artifacts, such as compound auto fluorescence and cytotoxicity. These datasets can aid in the identification of previously uncharacterized toxicants as well as the development of computational models for toxicity prediction. In this session, a data processing method will be described that evaluates the qHTS data quality and integrates signals from various assay readouts and counter screens to minimize biological and technological artifacts and produce a final call on on-target compound activity. Example applications of the Tox21 in vitro assay data to developing machine learning models for the prediction of in vivo and in vitro toxicity endpoints will be discussed. A demonstration of a detailed model construction process will be given.